Just as the Industrial Revolution marked a significant shift in history by introducing machines capable of tasks once thought only possible for humans, we are now entering a new transformative era with the rise of artificial intelligence (AI). At the forefront of this revolution is image recognition technology, which, at first glance, seems to mimic human perception. However, this initial impression belies a deeper, more fundamental difference in operation.
In reality, machine perception is distinct and unique. Machines have computational abilities far surpassing those of humans, enabling them to process information—specifically pixels—at a speed and efficiency thousands of times greater than our own.
The remarkable capacity of image recognition technology to detect patterns and make decisions with greater speed and efficacy than human capabilities is a key driver of its rapid market growth. According to industry forecasts, the global image recognition market is poised for a Compound Annual Growth Rate (CAGR) of 10.42% from 2023 to 2030, reflecting the increasing reliance on and confidence in this technology across multiple sectors.
Its application is becoming increasingly critical in fields such as healthcare, where it aids in diagnosing diseases with higher accuracy; in manufacturing, for optimizing quality control processes; in agribusiness, by monitoring crop health and enhancing yield predictions; and in transportation, particularly in the development of autonomous vehicles that require precise environmental understanding to navigate safely.
It’s crucial to understand that while image recognition technology may appear to emulate human perception, it fundamentally solves problems differently. This technology is already finding significant applications in the real world, despite being in its early stages, and holds vast potential for the future.
Understanding Image Recognition and Its Advantages
Image recognition technology allows computers to identify and classify objects within images, akin to human vision but with significantly greater speed and accuracy.
The primary advantage of image recognition lies in its ability to automate and enhance tasks that require visual identification, such as diagnosing diseases from medical images, improving security through facial recognition, and even optimizing agricultural practices by monitoring crop health.
How Image Recognition Works
The backbone of image recognition is Convolutional Neural Networks (CNNs), a type of deep learning algorithm modeled after the human brain’s visual cortex. CNNs process layers of an image to identify patterns and features, such as edges and textures, gradually recognizing more complex elements like shapes and objects.
This layered approach enables machines to perceive and interpret visual information efficiently.
Training Algorithms and the Issue of Bias
Training image recognition algorithms involves feeding them vast datasets of labeled images. These datasets allow algorithms to learn from examples and improve their ability to recognize similar objects in new images. However, these algorithms can inherit biases present in their training data, leading to skewed or unfair outcomes.
Addressing biases in CNNs for image recognition involves a multi-faceted approach to ensure fairness, accuracy, and reliability in AI applications. Here are some strategies:
- Diverse and Representative Training Data: Start by assembling datasets that accurately reflect the diversity of the real world. This involves not only including images from a wide range of demographics but also ensuring that the contexts and scenarios depicted in the images are varied and inclusive.
- Comprehensive Data Labeling: Implement detailed, bias-aware guidelines for data labeling. Labelers from diverse backgrounds can help ensure a broader perspective is applied to the task, reducing the risk of single-perspective bias.
- Bias Detection and Mitigation Tools: Techniques such as adversarial training can help identify and reduce biases by challenging the model to perform well across a diverse range of data.
- Transparent and Explainable AI (XAI): Implement XAI methods to make the model’s decision-making process transparent. This can help identify where and how biases might be influencing outcomes, providing an opportunity for targeted adjustments.
- Ethical Guidelines and Standards: Adhere to ethical guidelines that emphasize fairness and bias mitigation in AI. These standards can serve as a benchmark for developing, testing, and deploying AI systems responsibly.
- User Feedback Loops: Incorporate feedback from users, especially those from underrepresented groups, to gain insights into how the model’s outputs affect different demographics.
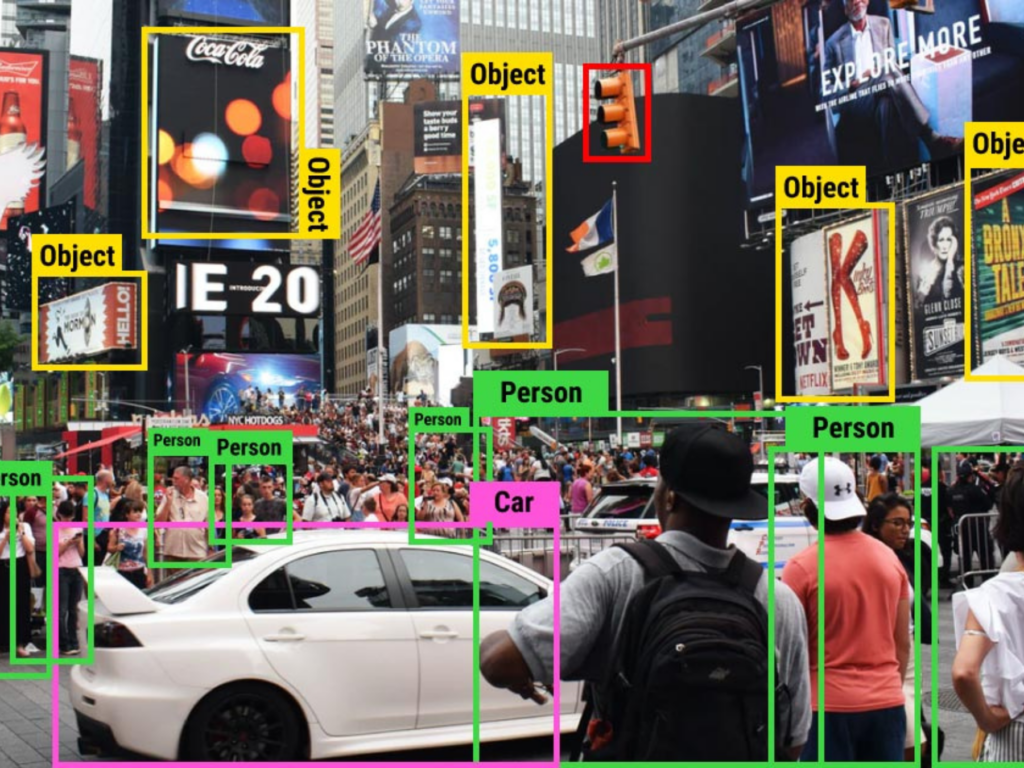
Machine vs. Human Perception: What Are the Differences?
Understanding the distinctions between machine and human perception is vital in grasping the capabilities and limitations of AI in tasks like image recognition.
Human perception is deeply contextual and enriched by personal experiences, emotions, and cultural backgrounds, enabling us to interpret visual stimuli beyond mere pattern recognition. For instance, our recognition of a friend’s face is interwoven with memories and emotions, adding layers of meaning that machines cannot replicate.
Machine perception, on the other hand, is purely data-driven. It lacks the human capacity for contextual understanding and emotional connection. Machines rely heavily on vast datasets to learn, and their recognition abilities are confined to the patterns and features explicitly present in this data.
Unlike humans, machines cannot extrapolate from limited examples or adapt their understanding based on subtle contextual clues. This fundamental difference often leads to errors that seem counterintuitive to human observers, such as misidentifying objects due to unusual backgrounds or lighting conditions that would not typically confuse a human.
A common misconception is to anthropomorphize machine perception, attributing human-like understanding and adaptability to AI systems. This overestimation can lead to overreliance on technology in sensitive areas, ignoring the potential for errors and biases inherent in current AI models.
Recognizing these differences is crucial for the responsible development and application of image recognition technologies, ensuring they are used as effective tools rather than infallible solutions.
Types of Algorithms for Pattern Recognition
Beyond CNNs, there are several algorithms key to enabling machines to perceive and recognize patterns:
- Support Vector Machines (SVMs): Used for classification tasks, SVMs can categorize images by distinguishing between object types based on learned differences.
- Recurrent Neural Networks (RNNs): Particularly useful for sequences, RNNs can recognize temporal patterns in video footage.
- Autoencoders: These are used for unsupervised learning, helping in feature detection and reduction to better understand and classify image content.
Real-World Applications Beyond Case Studies
1. Drones & AI Redefine Power Line Inspections
At Inclusion Cloud, we had the opportunity to partner with a leading energy company, for a transformative project related to energy transmission.
The primary challenge was to monitor over 20,000 km of transmission lines across an area of 2.78 million km². We understood that even a millisecond of energy disruption was unacceptable, given that these lines are the backbone for over 44 million people.
Our task was to enhance the inspection of extensive electric transmission lines. This meant improving safety, and efficiency, and ensuring an uninterrupted energy supply.
We developed a drone application integrated with image recognition to streamline the inspection process while ensuring the safety of the operators and the integrity of the power network. The drones, equipped with high-resolution cameras, systematically capture detailed images of the electric towers. These images are then analyzed by our advanced AI-driven image recognition system, which is trained to identify anomalies and signs of wear or damage that might not be immediately visible to human inspectors.
The results of implementing image recognition technology were significant. We achieved a 47% reduction in work-related incidents and integrated the application with SAP, leading to automated repair work orders. This not only increased productivity by 35% but also made the inspection process 90% safer.
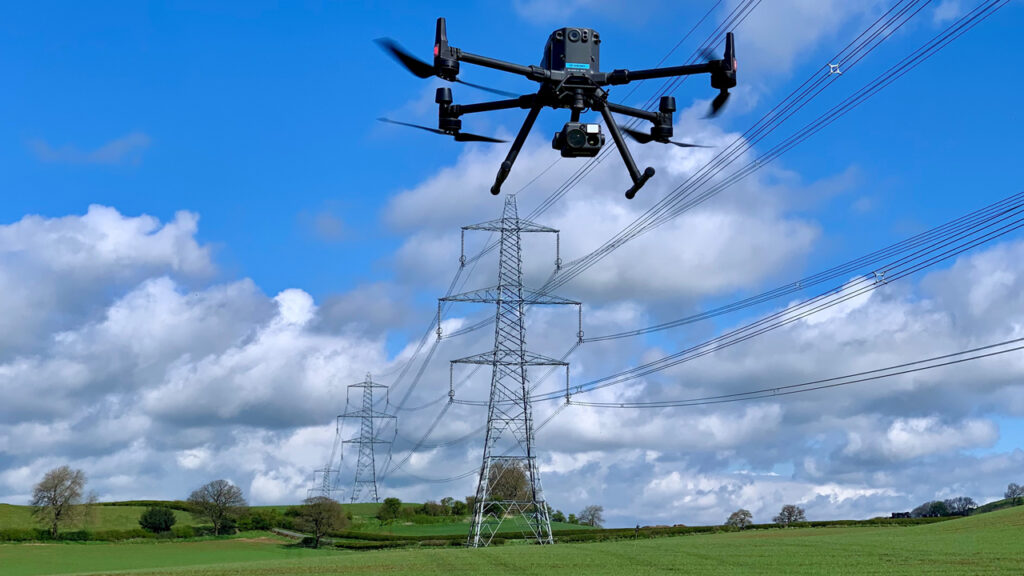
2. Transforming the Manufacturing Sector
Manufacturing steel pipes demands the utmost precision. In pursuit of a novel solution to streamline the extensive inventory processes of steel tubes within their warehouses, a leading company in the steel industry embarked on a transformative journey with the expertise of Inclusion Cloud’s talents. The breakthrough came with the integration of image recognition technology into mobile devices actively used on-site.
This advancement allowed the team to effortlessly capture images of the inventory, which were immediately analyzed to identify each steel tube and its corresponding QR code accurately. By seamlessly connecting this innovative system with the company’s Oracle ERP system, the collaboration not only enhanced detailed inventory management but also significantly reduced the potential for manual errors, showcasing the impactful synergy between industry knowledge and technological expertise.
3. Agricultural Innovations
In the sun-drenched agave fields of Mexico, a top tequila manufacturer has partnered with us to redefine traditional cultivation methods with a forward-thinking approach. Drones, armed with the latest in image recognition technology, soar above the green expanse, capturing precise details of the agave plants.
These images are processed through advanced software that carefully evaluates key indicators of plant health, including age, hydration, and growth patterns. This method not only improves the accuracy of plant monitoring but also provides a deeper analysis, fostering smarter farming decisions and superior crop management.
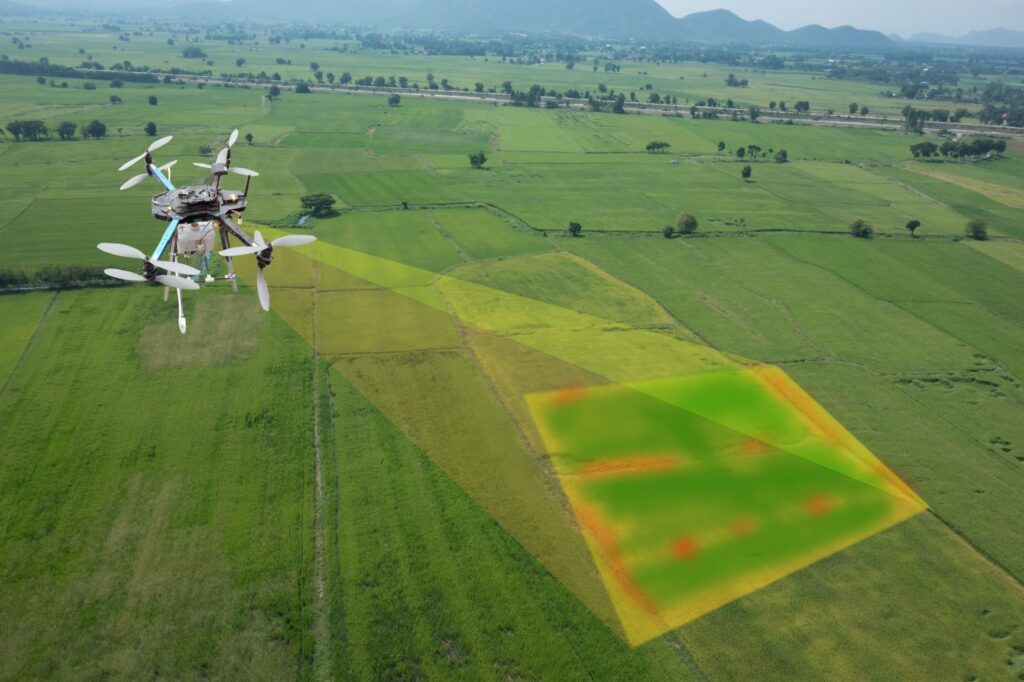
4. Advancements in Autonomous Vehicles
The journey of self-driving cars has had its ups and downs, stirring quite a bit of debate along the way. However, we’re seeing a bright future ahead, largely thanks to new developments in how these cars see the world around them. The key lies in image recognition technology, which allows cars to quickly and accurately make sense of their surroundings.
A standout advancement comes from MIT and the MIT-IBM Watson AI Lab, where researchers developed a model called EfficientViT. This model improves the car’s ability to identify everything around it, from other cars to pedestrians, by analyzing every detail in the images it captures.
This means self-driving cars can now process visual information better and faster, overcoming previous hurdles. These improvements don’t just make self-driving cars safer and more reliable; they also highlight the potential for these vehicles to change how we think about transportation in the future.
5. Medical Diagnostics Leap Forward
The healthcare sector is witnessing remarkable advancements through image recognition technology, exemplified by systems like Google’s Med Palm 2.
This cutting-edge platform represents a significant step forward in patient diagnostics, enabling the analysis of medical imagery with unparalleled speed and precision. Through sophisticated algorithms, it assists healthcare professionals in diagnosing a broad spectrum of conditions more effectively, contributing to enhanced patient care and outcomes.
This integration of image recognition and AI in healthcare exemplifies the promising convergence of technology and medicine for better, more reliable diagnostics.
The Future of Image Recognition
Image recognition technology is making a significant difference, making our devices smarter and allowing us to complete jobs more efficiently. It’s particularly adept at detecting problems that we would miss, and it’s a game changer for dangerous or difficult tasks like checking ships or wind turbines.
This technology is about more than simply speed; it’s also about safety and efficiency. It helps farmers monitor their fields and doctors detect diseases early. Image recognition is here to change how we tackle everyday tasks and bigger challenges.
Want to keep up with how image recognition is shaping the future? Follow us on LinkedIn. If you think this tech could help in your work, let’s chat. We’ve seen it make a real difference in efficiency, safety, and quality in various projects.